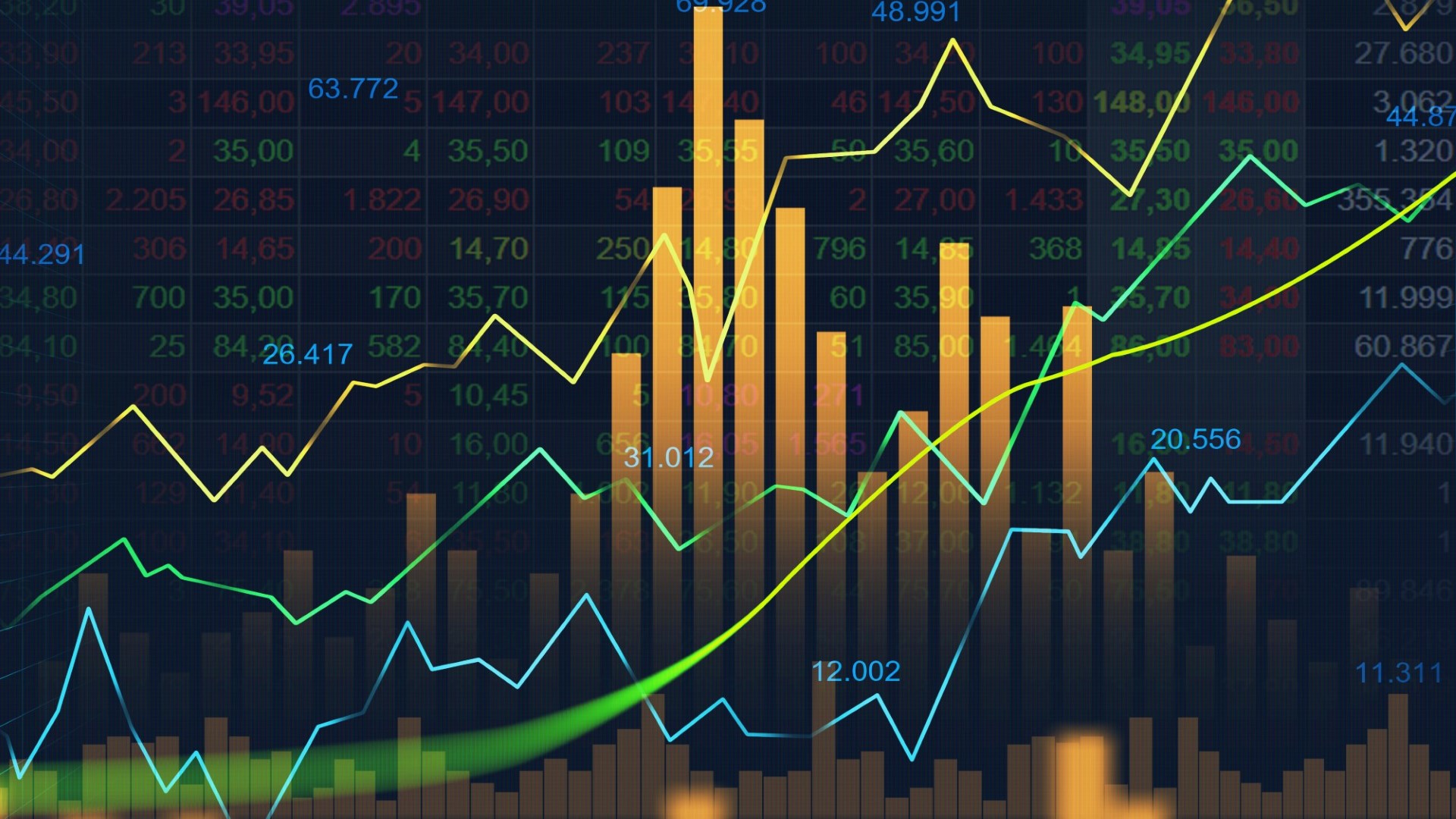
The buy side sees the U.S. Treasury market as largely transparent. That’s a good thing. However, less than half of that same group told us they regularly perform transaction cost analysis (TCA). That suggests one of two things: Either the buy side does not have as much visibility into the market as they think, or they’re not utilizing the transparency they do have as much as they should. Either way, it leaves me struggling to not restate one of the biggest clichés in business: “If you can’t measure it, you can’t improve it.”
Source: Coalition Greenwich 2021 Market Structure & Trading Technology Study.
To further that point, the existence of transparent markets does not guarantee execution quality. Executing at the price on the screen when the order was initiated could be good enough for some. Achieving the price target set by the portfolio manager or pricing models might be good enough for others. But truly achieving the best execution possible requires not simply measuring the resulting price against a sometimes arbitrary benchmark, but digging into the details trade after trade to ensure all of the right factors—time, size, price, objectives, market conditions, et al—are taken into account.
Reliable pre-trade market data acts as the fuel for execution algorithms, whether fully automated order-book-focused algorithms or those used to interact automatically in the request-for-quote (RFQ) market. In an ideal world, this includes full depth of book, which is readily available for U.S. Treasuries and U.S. Treasury futures, and historical market data going back minutes, months or years.
When the trading algorithms are done doing their thing, the pre-trade data can be combined with the resulting execution data to analyze what actually happened, whether the trade objectives were achieved and, mostly importantly, what could be done better next time. This could include information on better timing of that particular trade, an algorithm that might have been more effective or suggestions about which dealers might have been better suited to that trade. Quantitative Brokers provided us with this great example of TCA output they created for a 20-year U.S. Treasury trade, which notes a savings of $39.84 per lot traded:
These actionable results should then feed back into the pre-trade process, which starts the whole cycle again. The longer this goes on, the smarter the entire process should become, and hopefully, the better the execution quality received.
This process is not unlike the feedback loop seen in TCA products focused on U.S. equities, where TCA is effectively ubiquitous on the buy side. But is it important to reiterate that the U.S. Treasury market is not the U.S. equity market. Sure, the market is liquid and seen by investors as transparent, but bond-trading venues are much more lightly regulated, there is no trading tape (U.S. Treasury reporting is weekly, and in aggregate), and there is no retail presence to speak of. So while the high-level TCA process feels the same, the underlying mechanics of that process are vastly different.
First, trading in U.S. Treasuries happens across six major platforms, not including the third of the market that is handled via phone and instant message. Each platform has its own approach to normalizing and disseminating data, which means that outside of the largest banks and buy-side firms, few have access to the data offered by every platform, leading to an incomplete picture of the market. Even with the data in hand, the data licenses can often limit how that data is used.
Second, about 70% of trading activity in this market is done on a name-disclosed basis, with nearly half happening via RFQ. That means that a visible price is not necessarily the best price a trader can receive. Relationships matter with regards to pricing, and market makers often do not show their best prices in the anonymous market, saving those for direct-client interactions.
Lastly, the process is still largely a post-trade one at this point. Few investors told us they value TCA for its ability to help them pre-trade, which leaves us believing there remains a huge opportunity to move the market forward with enhanced pre-trade analytics.
Source: Coalition Greenwich 2021 Market Structure & Trading Technology Study.
In the late 1990s before fintech was cool, the technology available could not solve all of the business problems being put forth. Thirty years later, the tables have turned, with technology now able to handle tasks and challenges that some in the business aren’t interested in automating yet. One of the longest running roadblocks to TCA adoption is the perfect case in point—traders do not always want their performance to be measured!
Looking forward, TCA isn’t just for the buy side. Liquidity providers can and increasingly are measuring their performance in the market more precisely than ever. Dealer-to-client e-trading has grown over the past year, and with it, the amount of data available to market participants to measure their success. And if the SEC and FINRA move forward with proposed plans to enhance oversight and require more detailed reporting, the information available to all in the market should only increase.
The Coalition Greenwich 2021 Market Structure and Trading Technology Study is based on responses from 157 fixed-income investors in the U.S. and Europe. Asset managers made up 44% of responses, with the remainder a mix of hedge funds, insurance companies, regional banks, and others.